Programme des journées
Cette page fournit le programme de la journée publique du 4 mai. Elle sera mise à jour régulièrement.
Déroulé de la journée:
- 10h-11h: Leandro Nascimento, "Estimation d'erreur dans les images IRM"
- 11h-11h15: Pause café
- 11h15-12h15: Roman Malinowski, "incertitudes dans la reconstruction d'image 3D stéréoscopiques"
- 12h15-13h30: Pause repas
- 13h30-14h30: Claire Theobald, "A Bayesian Convolutional Neural Network for Robust Galaxy Ellipticity Regression"
- 14h30-15h30: Olivier Strauss, "imprécision et transformations géométriques"
- 15h30-15h45: Pause café
- 15h45-16h45: Luc Jaulin, "Detection and capture of intruders using robots"
- 16h45-17h45: Xuanlong Yu, "Towards scalable uncertainty estimation with deterministic methods, and their fair evaluation"
Lien zoom: https://utc-fr.zoom.us/j/83243567133
Détails des exposé prévus:
- Luc Jaulin: Detection and capture of intruders using robots
Résumé: The talk is motivated by the detection of submarine intruders inside the Bay of Biscay (golfe de Gascogne). In this project, we consider a group of underwater robots inside a zone with a known map (represented by an image). We assume that each robot is able to detect any intruder nearby. Moreover, the speed of the intruders is assumed to be bounded and is small with respect to that of our robots. The goal of this presentation is twofold: (1) to characterize an image representing the secure zone, i.e., the area for which we can guarantee that there is no intruder (2) to find a strategy for the group of robots in order to extend the secure zone as much as possible.
- Xuanlong Yu: Towards scalable uncertainty estimation with deterministic methods, and their fair evaluation
Résumé: Predictive uncertainty estimation is essential for deploying Deep Neural Networks in real-world autonomous systems. However, most successful approaches are computationally intensive. Recently proposed Deterministic Uncertainty Methods (DUM) can only partially meet such requirements as their scalability to complex computer vision tasks is not obvious. In this presentation, we first introduce a scalable and effective DUM for high-resolution semantic segmentation, that relaxes the Lipschitz constraint typically hindering practicality of such architectures. We learn a discriminant latent space by leveraging a distinction maximization layer over an arbitrarily-sized set of trainable prototypes. Our approach achieves competitive results over Deep Ensembles, the state of the art for uncertainty prediction, on image classification, segmentation and monocular depth estimation tasks.
In terms of evaluation, we will highlight the challenges raised by autonomous driving perception tasks, for which disentangling the different types and sources of uncertainty is non-trivial for most datasets, especially since there is no ground truth for uncertainty. In addition, while adverse weather conditions of varying intensities can disrupt neural network predictions, they are usually under-represented in both training and test sets in public datasets. We introduce the MUAD dataset (Multiple Uncertainties for Autonomous Driving), consisting of 10,413 realistic synthetic images with diverse adverse weather conditions (night, fog, rain, snow), out-of-distribution objects, and annotations for semantic segmentation, depth estimation, object and instance detection. MUAD allows to better assess the impact of different sources of uncertainty on model performance. We conduct a thorough experimental study of this impact on several baseline Deep Neural Networks across multiple tasks, and release our dataset to allow researchers to benchmark their algorithm methodically in adverse conditions.
- Roman Malinowski: incertitudes dans la reconstruction d'image 3D stéréoscopiques
Résumé: Photogrammetry, or reconstructing 3D models from a pair of left-right images, is a complex task with numerous processing steps. One of the most crucial step, dense matching, consists of finding the displacement of every pixel between the left image and the right image. It usually is the major source of error in a 3D pipeline. This talk intends to present methods used to propagate and quantify uncertainty in a dense matching problem. A concrete example of uncertainty propagation in a dense matching application will be presented. It considers uncertain images whose radiometry is modeled by possibility distributions. We use copulas as dependency models between variables and propagate the imprecise models. The propagation steps are detailed in the simple case of the Sum of Absolute Difference cost function for didactic purposes, and validated using Monte Carlo simulations. We will also present ideas on how to deduce confidence intervals for the displacement of pixels.
- Claire Theobald: A Bayesian Convolutional Neural Network for Robust Galaxy Ellipticity Regression
Résumé: Cosmic shear estimation is an essential scientific goal for large galaxy surveys. It refers to the coherent distortion of distant galaxy images due to weak gravitational lensing along the line of sight. It can be used as a tracer of the matter distribution in the Universe. The unbiased estimation of the local value of the cosmic shear can be obtained via Bayesian analysis which relies on robust estimation of the galaxies ellipticity (shape) posterior distribution. This is not a simple problem as, among other things, the images may be corrupted with strong background noise. For current and coming surveys, another central issue in galaxy shape determination is the treatment of statistically dominant overlapping (blended) objects. We propose a Bayesian Convolutional Neural Network based on Monte-Carlo Dropout to reliably estimate the ellipticity of galaxies and the corresponding measurement uncertainties. We show that while a convolutional network can be trained to correctly estimate well calibrated aleatoric uncertainty, -the uncertainty due to the presence of noise in the images- it is unable to generate a trustworthy ellipticity distribution when exposed to previously unseen data (i.e. here, blended scenes). By introducing a Bayesian Neural Network, we show how to reliably estimate the posterior predictive distribution of ellipticities along with robust estimation of epistemic uncertainties. Experiments also show that epistemic uncertainty can detect inconsistent predictions due to unknown blended scenes.
- Olivier Strauss: imprécision et transformations géométriques
Résumé: Digital image processing has become the most common form of image processing. A wide branch of image processing algorithms make an extensive use of spatial transformations that are only defined in the continuous domain such as rotation, translation, zoom, anamorphosis, homography, distortion, derivation, etc.
Designing a digital image processing algorithm that mimic a spatial transformation is usually achieved by using the so-called kernel based approach. This approach involves two kernels to ensure the continuous to discrete interplay: the sampling kernel and the reconstruction kernel, whose choice is highly arbitrarily made.
In this talk, we propose the maxitive kernel based approach as an extension of the conventional kernel based approach that reduces the impact of such an arbitrary choice. Replacing a conventional kernel by a maxitive kernel in a digital image spatial transformation leads to compute the convex set of all the images that would have been obtained by using a (continuous convex set) of conventional kernels. Using this set induces a kind of robustness that can reduce the risk of false interpretation.
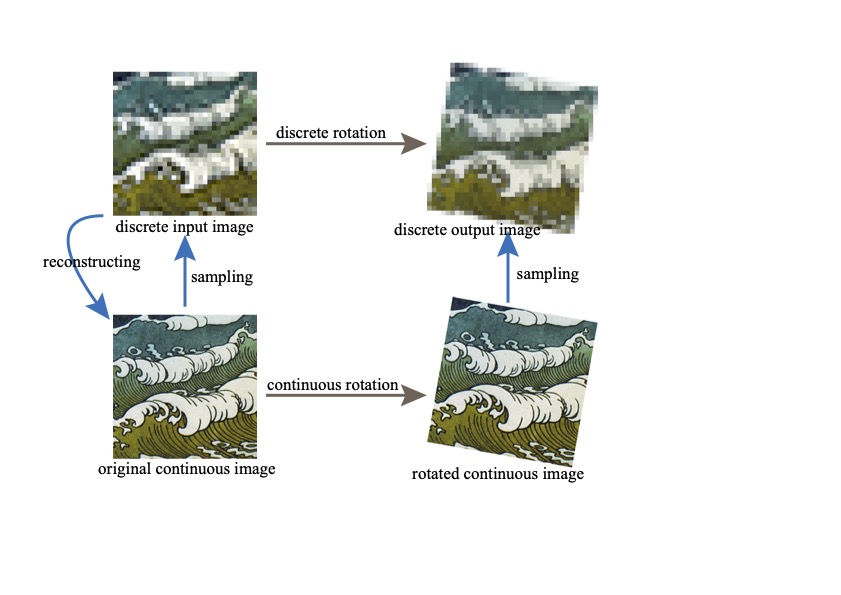
- Leandro Nascimento: Estimation d'erreur dans les images IRM
Résumé: Les distorsions des images par résonance magnétique (IRM) et le recalage de l'IRM sur la tomodensitométrie (TDM - CT scan) sont deux des principales sources d'erreur dans le cadre des chirurgies stéréotaxiques cérébrales. L’efficacité de plusieurs chirurgies cérébrales clés, telles que la stimulation cérébrale profonde (SCP), dépend aujourd'hui directement de la précision stéréotaxique. Cependant, l'estimation de l'erreur de recalage (registration error estimation - REE - en anglais) est une tâche difficile à cause de l'absence de vérité terrain. Pour résoudre ce problème, nous proposons un modèle U-Net de régression capable d'estimer les erreurs de recalage. Nos expériences sur le recalage mono-modal IRM ont fourni des résultats préliminaires comparables à ceux des travaux similaires précédents.
|